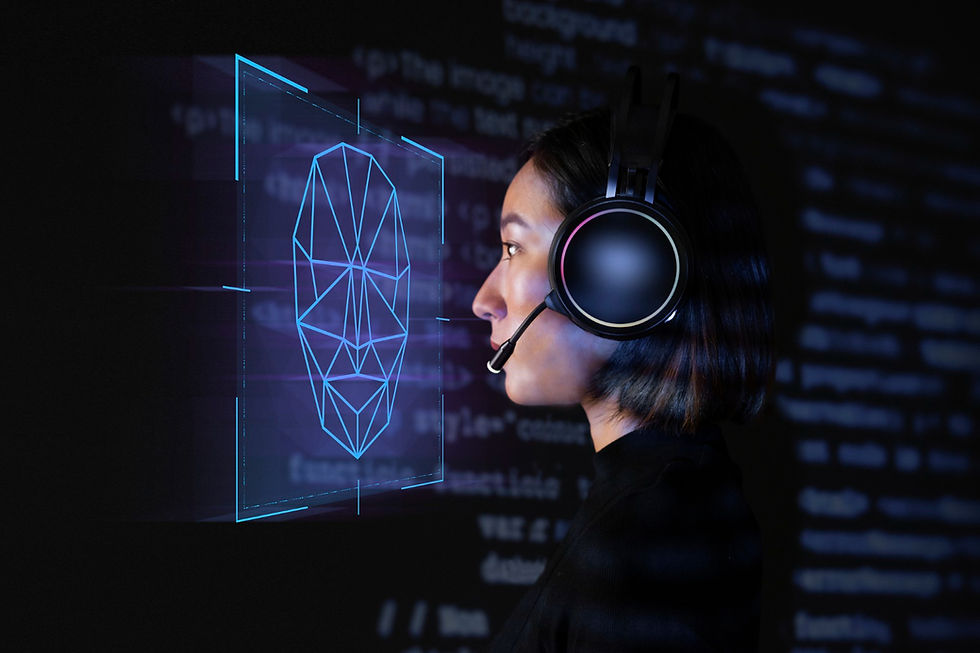
Artificial intelligence stands as one of the most prominent buzzwords in the tech sphere,
and rightfully so. AI and generative AI are revolutionizing the IT landscape by
streamlining tasks that were once considered hard to perform.
Since the release of ChatGPT in late 2022, usage of artificial intelligence (AI) in various
fields had considerably grown. This is fundamentally reshaping the landscape of various
fields, including cyber security. AI will surely change the rules of the game.
Although how AI will impact cyber security is yet to known, it sure offers some benefits.
It can help detect, analyze, and respond to malicious attacks faster. AI-based cyber
security systems provide improved accuracy and efficiency compared to traditional
security solutions. AI can automate tedious security tasks, freeing valuable resources to
focus on other business areas, reducing response times to security incidents and helps
lower the cost of defending against cyber threats.
But, the Sober Truth? Apart from the said benefits, more and more attacks will utilize AI.
It's an undeniable reality that AI-driven attacks are becoming increasingly prevalent. In
2023, we saw broad adoption of Large Language Models (LLMs). Large Language Models
(LLMs) are actively reshaping the cybersecurity landscape, introducing transformative
changes. Nonetheless, they also pose unprecedented challenges.
The Risks of Relying on AI in Cyber Security
Cyber attackers are leveraging AI capabilities to engineer malware that dynamically
adapts, evolves, and learns from its surroundings. This sophisticated AI-driven malware
is designed to circumvent traditional security measures, constantly morphing its
behaviour to evade signature-based detection systems and exploit vulnerabilities with
unparalleled agility.
Like this, there are also significant number of extra risks associated with relying solely
on AI for cybersecurity:
1.Adversarial Attacks: AI systems are susceptible to adversarial attacks, where malicious
actors manipulate input data to deceive AI algorithms. Exploiting vulnerabilities in AI
models can enable attackers to bypass detection mechanisms, leading to false positives
or negatives and undermining the overall effectiveness of cybersecurity defenses.
2.Bias and Discrimination in Decision-Making: AI models trained on biased data may
perpetuate existing biases and discrimination. In the realm of cybersecurity, biased AI
algorithms could potentially overlook threats targeting specific demographics or
regions, resulting in unequal protection and leaving security vulnerabilities
unaddressed.
3.Data Privacy Concerns: AIdriven cybersecurity involves the analysis of vast amounts of sensitive data. Mishandling or unauthorized access to this data poses significant privacy risks, potentially resulting in regulatory non-compliance and privacy violations that could tarnish an organization's reputation.
4.Overdependence and Complacency: Excessive reliance on AI systems may lead to
complacency among cybersecurity professionals. Overconfidence in AI's capabilities
could result in overlooking critical security alerts or failing to intervene when necessary,
assuming that AI will handle all aspects of threat detection and response.
5.Lack of Explainability and Transparency: AI systems lack the contextual
understanding and nuanced reasoning abilities of humans. They may misinterpret
benign activities as malicious or fail to recognize sophisticated, context-dependent
attacks, leading to inaccurate threat assessments and potentially costly false alarms.
6.Dependency on Training Data Quality: The effectiveness of AI models in
cybersecurity heavily depends on the quality and representativeness of the training
data. Incomplete, biased, or outdated training data can compromise the accuracy and
reliability of AI-driven security solutions, leaving organizations vulnerable to emerging
and evolving threats.
7.Regulatory and Ethical Challenges: The use of AI in cybersecurity raises complex
regulatory and ethical considerations. Organizations must navigate regulatory
frameworks and ensure that AI systems adhere to ethical principles and legal
requirements, including accountability, transparency, and fairness, to maintain trust and
integrity in their cybersecurity practices.
When implementing AI solutions in business processes especially security, it's crucial to
consider several key factors:
Top Points to consider While implementing AI solutions in
Cybersecurity
Data Quality: Ensure that you have high-quality, relevant data that is properly labelled and structured for training your AI model effectively.
Model Selection: Choose the appropriate AI model based on the nature of the problem, available data, and desired level of accuracy. Consider factors like deep learning, machine learning, or other specialized algorithms.
Hardware and Infrastructure: Assess the computational resources needed to train and deploy your AI model. Ensure that you have the necessary hardware infrastructure or access to cloud services that can support your requirements.
Explainability: Strive for transparency in your AI model's decision-making process, particularly in critical domains like healthcare or finance. Understandability and interpretability are essential for building trust and meeting regulatory requirements.
Security and Privacy: Protect sensitive data throughout the AI lifecycle, including during data collection, model training, deployment, and inference. Implement robust security measures to prevent unauthorized access or data breaches.
To mitigate these risks effectively, organizations must adopt a holistic approach to
cybersecurity that combines AI-driven tools with human expertise, robust data
governance practices, continuous monitoring, and evaluation, and proactive measures to
address emerging threats. Additionally, promoting transparency and explainability in AI
algorithms can enhance trust and facilitate informed decision-making in cybersecurity
operations.
תגובות